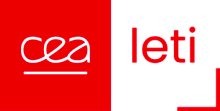
Breakthrough Classifies Types of Arrhythmia Recordings With Precise Aleatoric
and Epistemic Uncertainty
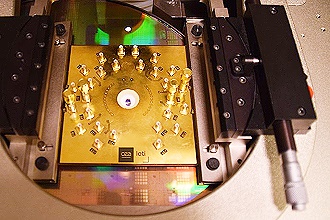
Wafer-level characterization of memristor crossbar arrays using
a prober, highlighting detailed probe card analysis at an enlarged scale.
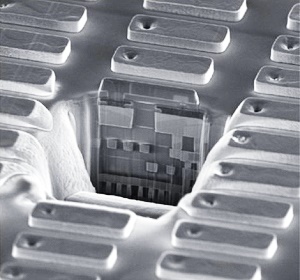
Scanning Electron Microscopy image of a filamentary memristor in the back end
of line of our hybrid memristor/CMOS process.
Grenoble, France - Dec. 7, 2023 - Considering medical-diagnosis and other safety-critical,
sensory-processing applications that require accurate decisions based on a small
amount of noisy input data, the study notes that while Bayesian neural networks
excel at such tasks because they provide predictive uncertainty assessment, their
probabilistic nature requires increased use of energy and computation.
The increase is caused by the fact that implementing the networks in hardware
requires a random number generator to store the probability distributions, i.e.
synaptic weights.
"Our paper presents, for the first time, a complete hardware implementation of
a Bayesian neural network utilizing the intrinsic variability of memristors to store
these probability distributions," said Elisa Vianello, CEA-Leti chief scientist.
"We exploited the intrinsic variability of memristors to store these probability
distributions, instead of using random number generators."
A team comprising CEA-Leti, CEA-List and two CNRS laboratories has published
a new paper in Nature Communications presenting the first complete memristor-based
Bayesian neural network implementation for a real-world taskâclassifying types of
arrhythmia recordings with precise aleatoric and epistemic uncertainty.
Considering medical-diagnosis and other safety-critical, sensory-processing applications
that require accurate decisions based on a small amount of noisy input data, the
study notes that while Bayesian neural networks excel at such tasks because they
provide predictive uncertainty assessment, their probabilistic nature requires increased
use of energy and computation. The increase is caused by the fact that implementing
the networks in hardware requires a random number generator to store the probability
distributions, i.e. synaptic weights.
"Our paper presents, for the first time, a complete hardware implementation of
a Bayesian neural network utilizing the intrinsic variability of memristors to store
these probability distributions," said Elisa Vianello, CEA-Leti chief scientist
and co-author of the paper, "Bringing Uncertainty Quantification to the Extreme-Edge
with Memristor-Based Bayesian Neural Networks". "We exploited the intrinsic variability
of memristors to store these probability distributions, instead of using random
number generators."
A second major challenge was that performing inference in the team's approach
requires massive parallel multiply-and-accumulate (MAC) operations.
"These operations are power-hungry when carried out on CMOS-based ASICs and field-programmable
gate arrays, due to the shuttling of data between processor and memory," Vianello
said. "In our solution, we use crossbars of memristors that naturally implement
the multiplication between the input voltage and the probabilistic synaptic weight
through Ohm's law, and the accumulation through Kirchhoff's current law, to significantly
lower power consumption."
Reconciling Memristors and Bayesian Neural Networks
Co-author Damien Querlioz, a scientist associated with the University of Paris-Saclay,
the French National Center for Scientific Research (CNRS) and the Center of Nanosciences
and Nanotechnologies, said the team also had to reconcile the nature of memristors,
whose statistical effects adhere to the laws of device physics, with Bayesian neural
networks, in which these effects can take arbitrary shapes.
"This work overcomes that challenge with a new training algorithm - variational
inference augmented by a 'technological loss' - that accommodates device non-idealities
during the learning phase," he said. "Our approach enables the Bayesian neural network
to be compatible with the imperfections of our memristors."
Uncertainty quantification involves the network's ability to identify unknown
situations out-of-distributions.
If a traditional neural network trained to recognize cats and dogs is presented
with an image of a giraffe, it "confidently misclassifies" it as a cat or a dog,
Vianello said. "In contrast, a Bayesian neural network would respond, 'I am not
entirely sure what this is because I have never seen it.' While this example is
lighthearted, in critical environments like medical diagnosis, where incorrect predictions
can have severe consequences, this uncertainty-capturing ability becomes crucial."
This capability arises from the fact that synaptic values in Bayesian neural
networks are not precise values, as in traditional neural networks, but rather probability
distributions. Consequently, the output is also a probability distribution, providing
information about its "certainty".
Scanning Electron Microscopy image of a filamentary memristor in the back end
of line of our hybrid memristor/CMOS process
About CEA-Leti (France)
CEA-Leti, a technology research institute at CEA, is a global leader in miniaturization
technologies enabling smart, energy-efficient and secure solutions for industry.
Founded in 1967, CEA-Leti pioneers micro-& nanotechnologies, tailoring differentiating
applicative solutions for global companies, SMEs and startups. CEA-Leti tackles
critical challenges in healthcare, energy and digital migration. From sensors to
data processing and computing solutions, CEA-Leti's multidisciplinary teams deliver
solid expertise, leveraging world-class pre-industrialization facilities. With a
staff of more than 2,000 talents, a portfolio of 3,200 patents, 11,000 sq. meters
of cleanroom space and a clear IP policy, the institute is based in Grenoble, France,
and has offices in Silicon Valley, Brussels and Tokyo. CEA-Leti has launched 75
startups and is a member of the Carnot Institutes network. Follow us on www.leti-cea.com
and @CEA_Leti.
Technological expertise CEA has a key role in transferring scientific knowledge
and innovation from research to industry. This high-level technological research
is carried out in particular in electronic and integrated systems, from microscale
to nanoscale. It has a wide range of industrial applications in the fields of transport,
health, safety and telecommunications, contributing to the creation of high-quality
and competitive products.
For more information: www.cea.fr/english
Contact Info
Sarah-Lyle Dampoux
CEA-Leti Press Contact Agency
E-mail:
sldampoux@mahoneylyle.com
Phone: +33 6 74 93 23 47
Posted December 8, 2023
|